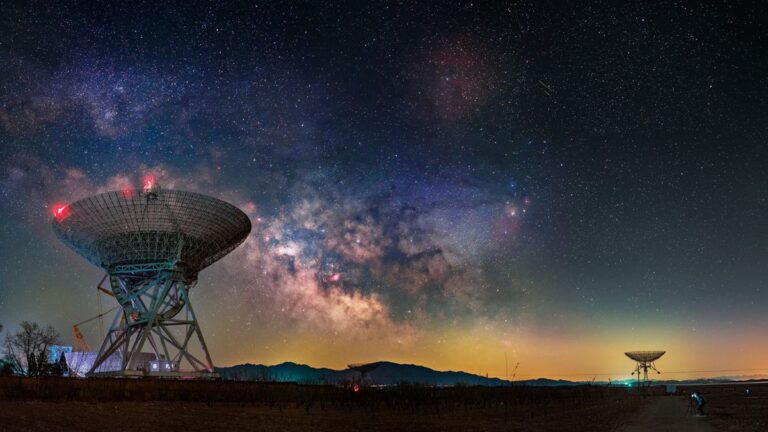
[ad_1]
If the search for extraterrestrial intelligence (SETI) is successful, we may require the help of artificial intelligence (AI) to understand what the aliens are saying and, perhaps, talk back to them.
In popular culture, we’ve gotten used to aliens speaking English, or being instantly understandable with the help of a seemingly magical universal translator. In real life, it might not be so easy.
Consider the potential problems. Number one would be that any potential aliens we encounter won’t be speaking a human language. Number two would be the lack of knowledge about the aliens’ culture or sociology — even if we could translate, we might not understand what relevance it has to their cultural touchstones.
Eamonn Kerins, an astrophysicist from the Jodrell Bank Centre for Astrophysics at the University of Manchester in the U.K., thinks that the aliens themselves might recognize these limitations and opt to do some of the heavy lifting for us by making their message as simple as possible.
“One might hope that aliens who want to establish contact might be attempting to make their signal as universally understandable as possible,” said Kerins in a Zoom interview. “Maybe it’s something as basic as a mathematical sequence, and already that conveys the one message that perhaps they hoped to send in the first place, which is that we’re here, you’re not alone.”
Related: Could AI find alien life faster than humans, and would it tell us?
Indeed, the possibility of receiving recognizable mathematical information — pi, a burst of prime numbers in sequence (as was the case in the novel “Contact” by Carl Sagan) — has been considered in SETI for decades, but it’s not the only possible message that we might receive. Other signals might be more sophisticated in their design, trying to convey more complicated concepts, and this is where we hit problem number three: That alien language could be orders of magnitude more complex than human communication.
This is where we will need AI’s help, but to understand how, first we must delve into the details behind the structure of language.
Information theory
When we talk about a signal or a message being complex, we don’t mean that the aliens will necessarily be talking about complex matters. Rather, it refers to the complexity underlying the structure of their message, their language. Linguists call this “information theory,” which was developed by the cryptographer and mathematician Claude Shannon who worked at Bell Labs in New Jersey in the late 1940s, and was expanded on by linguist George Zipf of Harvard University.
Information theory is a way of distilling the information content of any given communication. Shannon realized that any kind of conveyance of information — be it human language, the chemical exhalations of plants to attract predators to eat caterpillars on their leaves or the transmission of data down a fiber optic cable — can be broken down into discrete units, or bits. These are like the ‘quanta’ of communication, such as the letters of the alphabet or a dolphin’s repertoire of whistles.
In language, these bits cannot just go in any order. There is syntax, which describes the grammatical rules that dictate how the bits can be ordered. For example: In English, a ‘q’ at the beginning of a word is always followed by a ‘u’, and then the ‘u’ can be followed by a limited number of letters, and so on. Now suppose there is a gap — ‘qu—–k’. We know from the syntax that there are only a few combinations of letters that can fill the gap — ‘ac’ (quack), ‘ar’ (quark), ‘ic’ (quick) and ir (quirk). But, if the word is part of a sentence — ‘The duck went qu––k’ then through context we know the missing letters are ‘ac’.
By knowing the rules, or syntax, we can fill in the blanks. The amount missing that still allows us to complete the word of sentence is called “Shannon entropy,” and thanks to its complexity, human languages have the highest Shannon entropy of any known form natural communication on the planet.
Meanwhile, Zipf was able to quantify these basic principles of Shannon’s information theory. In any communication some of the little units, these fundamental bits, will appear more often than others. For example, in human language, letters such as a e, o, t and r appear far more often than q or z. When plotted on a graph with the most common units first (on the x-axis, their rate of occurrence on the y-axis), all human languages produce a slope with a gradient of –1. At the other extreme, a baby’s random babbling results in a horizontal line on the graph, with all sounds being equally likely. The more complex the communication — as the baby grows into a toddler and starts to talk, for example — the more the slope converges on a –1 gradient.
A transmission of the digits of pi, for instance, would now carry a –1 slope. So instead of searching for technosignatures, the technologically-generated signals that could mark other advanced extraterrestrial civilizations, some researchers think that SETI should be specifically looking for signals with a –1 slope, regardless of whether they appear artificial or not, and the machine-learning algorithms that carefully sift through every scrap of data collected by radio telescopes could be configured to analyze each potential signal to determine whether a signal adheres to Zipf’s Law.
Beyond that, alien communication could have a higher Shannon entropy than human language, and if it is much higher, it might make their language too difficult for humans to grasp.
But perhaps not for AI. Already, AI is being put to the test trying to understand communication from a non-human species. If it can pass that test, perhaps AI will be ready to tackle any alien messages in the future.
Interpreting dolphin communication
Denise Herzing, who is the Research Director at the Wild Dolphin Project in Jupiter, Florida, is one of the world’s foremost experts in trying to understand what dolphins are saying to each other. Herzing has been swimming with dolphins and studying their communication for four decades, and has now introduced AI into the mix.
“We have two ways in which we’re looking at dolphin communication, and they both use AI,” Herzing told Space.com.
One way is listening to recordings of the various whistles and barks that make up the dolphins’ own communication. In particular, a machine-learning algorithm is able to take a snippet of dolphin chat and break that communication down into discrete units on a spectrogram (a graph of sounds organized by frequency), just as Shannon and Zipf described, and then it labels each unique unit with a letter. These become analogous to words or letters, and Herzing is looking at the different ways they combine, or in other words their degree of order and structure.
“Right now we’ve identified 24 small units of sound that recombine within a spectrogram,” said Herzing. “So you might have up-whistle ‘A’ followed by down-whistle ‘B,’ and so on, and this creates a symbolic code for a sequence of sound.”
The machine-learning algorithm is then able to deeply analyze the sound recordings, searching for instances where that symbolic code is repeated.
“We’re looking for interesting sequences that are somehow repetitive,” said Herzing. “The algorithms then look for substitutions and deletions in the sequences, so you might have the same symbolic code but one little whistle is different. That’s a learning algorithm that is pretty important.”
That little difference could be because it incorporates a dolphin’s signature whistle (every dolphin has its own unique signature whistle, a kind of identifier like human names) or because the context is different.
This is all solidly in line with Shannon’s information theory, and Herzing is also interested in Zipf’s law and how closely dolphin communication replicates that –1 slope.
“We’re looking for language-like structures, because every language has a structure and a grammar that follows rules,” said Herzing. “We’re looking specifically for what the possibilities are for recombinational data — are our little units of sound only found alone, or do some recombine with another sound?”
Herzing’s team have been searching for bigrams — occasions when two units frequently occur together, which might signify a specific phrase. More recently, they have also been searching for trigrams — where three units occur in order regularly — implying greater complexity.
Searching for meaning
This is exactly the way that AI would begin analyzing a real message embedded within a SETI signal. If the alien communication is more complex in structure and syntax than human languages then that tells us something about them; perhaps that their species is older than our own, which has given them enough time for their communication to evolve.
However, we still wouldn’t know the context of what they are saying to us in the message. This is currently one of the challenges in understanding dolphin communication. Herzing has video footage of dolphin pods to see what they were doing whenever the AI detects a repeated vocalization of symbolic code, which allows Herzing to try and infer context to the sounds.
“But if you’re dealing with radio signals, how are you ever going to figure out what the context of the message is?” asks Herzing, who also takes an interest in SETI. “Looking at animal sounds is an analog for looking at alien signals, potentially to build up the tools to categorize and analyze [the signals]. But for the interpretation part? Oh boy, I don’t know.”
Once we have received a signal from aliens, we may want to say something back to them. The difficulty in understanding context rears its head again here, too. As Spock says in the film “Star Trek IV: The Voyage Home,” when discussing responding to an alien probe, “we could replicate the sounds but not the meaning. We’d be responding in gibberish.”
Herzing is trying to circumvent this context problem by mutually agreeing with the dolphins what to call things. This is the essence of CHAT (Cetacean Hearing and Telemetry), which is the second way in which researchers are using AI to try and communicate with dolphins.
In its first incarnation, CHAT was a large device strapped around the chest of the user, receiving sounds via hydrophone (underwater microphone) and then producing sound through a speaker. The modern version is smartphone-sized and worn around the wrist. The idea is not to converse in ‘dolphinese,’ but to agree with the dolphins upon pre-programmed sounds for certain toys that the dolphins want to play with. For example, if they want to play with a hoop, they make the agreed-upon whistle for ‘hoop’. If a diver wearing the CHAT device wants a dolphin to bring them a hoop, the underwater speaker can play the whistle for “hoop.” The AI’s job is to recognize the agreed-upon whistle amongst all the other sounds a dolphin makes amidst all the various sources of audio interference underwater, such as bubbles and boat propellers.
Herzing has observed that the dolphins have used the agreed-upon whistles, but in mostly different contexts. The problem, says Herzing, is spending enough time with any one particular dolphin to allow them to fully learn the agreed-upon sounds.
With aliens, their message will have traveled many light years; any two-way communication could take decades, centuries, millennia, if it is even possible at all. So whatever information we have about the aliens will be condensed into their original transmission. If, as Kerins suspects, they send something mathematical just as a signal to us that they are there and we are not alone, then we won’t have to worry about deciphering it.
However if they do send a message that is more involved, then as Herzing is discovering with dolphins, the size of the dataset is crucial, so let’s hope the aliens pack their message with information to give us and AI the best chance of at least assessing some of it.
[ad_2]