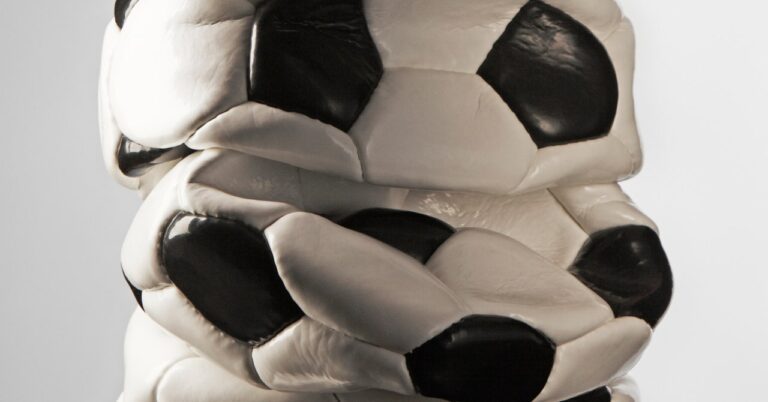
[ad_1]
“This didn’t actually work,” says Nicolas Heess, additionally a analysis scientist at DeepMind, and one of many paper’s coauthors with Lever. Due to the complexity of the issue, the large vary of choices obtainable, and the dearth of prior information in regards to the job, the brokers didn’t actually have any concept the place to start out—therefore the writhing and twitching.
So as an alternative, Heess, Lever, and colleagues used neural probabilistic motor primitives (NPMP), a educating methodology that nudged the AI mannequin in direction of extra human-like motion patterns, within the expectation that this underlying information would assist to unravel the issue of tips on how to transfer across the digital soccer pitch. “It mainly biases your motor management towards reasonable human conduct, reasonable human actions,” says Lever. “And that’s learnt from movement seize—on this case, human actors taking part in soccer.”
This “reconfigures the motion house,” Lever says. The brokers’ actions are already constrained by their humanlike our bodies and joints that may bend solely in sure methods, and being uncovered to knowledge from actual people constrains them additional, which helps simplify the issue. “It makes helpful issues extra prone to be found by trial and error,” Lever says. NPMP hastens the training course of. There’s a “delicate stability” to be struck between educating the AI to do issues the best way people do them, whereas additionally giving it sufficient freedom to find its personal options to issues—which can be extra environment friendly than those we give you ourselves.
Primary coaching was adopted by single-player drills: working, dribbling, and kicking the ball, mimicking the best way that people may study to play a brand new sport earlier than diving right into a full match state of affairs. The reinforcement studying rewards had been issues like efficiently following a goal with out the ball, or dribbling the ball near a goal. This curriculum of abilities was a pure method to construct towards more and more advanced duties, Lever says.
The intention was to encourage the brokers to reuse abilities they may have realized outdoors of the context of soccer inside a soccer surroundings—to generalize and be versatile at switching between totally different motion methods. The brokers that had mastered these drills had been used as academics. In the identical approach that the AI was inspired to imitate what it had realized from human movement seize, it was additionally rewarded for not deviating too removed from the methods the trainer brokers utilized in explicit situations, at the least at first. “That is truly a parameter of the algorithm which is optimized throughout coaching,” Lever says. “Over time they’ll in precept cut back their dependence on the academics.”
With their digital gamers skilled, it was time for some match motion: beginning with 2v2 and 3v3 video games to maximise the quantity of expertise the brokers gathered throughout every spherical of simulation (and mimicking how younger gamers begin off with small-sided video games in actual life). The highlights—which you’ll be able to watch right here—have the chaotic vitality of a canine chasing a ball within the park: gamers don’t a lot run as stumble ahead, perpetually on the verge of tumbling to the bottom. When objectives are scored, it’s not from intricate passing strikes, however hopeful punts upfield and foosball-like rebounds off the again wall.
[ad_2]